Arpit first worked on data at scale when he was the Head of Growth at Integromat (now Make), a workflow automation company. At the time, Integromate was organically acquiring a lot of users at a fast pace. Arpit and the team realized they needed to start driving growth using data to increase activation.
Arpit was the first go-to-market hire at Integromat. His efforts were focused toward building their user community and creating educational content for growth.
Their content and community efforts resulted in the acquisition of thousands of users but the problem was that the activation was pretty low:
"A lot of users would come to Integromat but not necessarily become active users because the product had a steep learning curve. That's when we decided to create a growth team and start using data to better understand what's going on, what users are doing inside the product, where they're getting stuck.
We also used the same data to personalize their experiences, offer more contextual in-app help, and deliver more contextual lifecycle emails."
After he moved on from Integromat, he started writing content about data for non-data people under different labels:
"I started writing for folks working in product growth and marketing, which I sometimes refer to as 'data adjacent' teams. I was really passionate about creating data content for folks like me who didn't really have a background in data or engineering."
These content creation efforts led him to create Data-led Academy, which was eventually acquired by Amplitude in 2022.
Arpit is now growing Databeats, a learning community and B2B media company where people can learn about data without necessarily having a data background.
The growth engine of Integromat
Because they were already acquiring a lot of users at the time, Integromat's goal for their growth engine was to set up three workflows:
- Product analytics
- Lifecycle emails
- In-app help, walkthroughs, and product tours
Product analytics
"This helped us understand where users were getting stuck in the product and where they're getting dropped."
Lifecycle emails
"We delivered lifecycle emails based on in-app events or things people were doing or not doing inside the app."
In-app help, walkthroughs, and product tours
"We also based these on user activity, user action, or events people were performing or not performing in the app."
Aside from deriving insights from their behavioral and event data, Integromat also used these data to run data-powered campaigns and experiments (now known as data activation).
Hyperfocus on activation
Integromat hyper focused on getting users to reach their first activation milestone, where they create their first scenario or integration in the app. The next goal was for the user to continually use the app:
"The goal was to get them to create more scenarios so that they would consume more operation, ultimately hit the limits of the free plan, and decide to upgrade their plan.
For that, we had a different workflow where we would share a lot of ideas. We would also collect a lot of demographic data and zero party data about our users as part of the onboarding process. This data helped us personalize our emails and messages based on the industry the user belonged to or their role in the company."
What is data-powered growth?
"It's simply using data, especially first-party data, to drive growth."
Data-powered growth has two parts: derivation of insights and data activation.
"You have the data, you derive some insights, and now you're activating the data. It could be in the form of creating experiments or campaigns to deliver personalized experiences.
At the same time, you're also making decisions based on the insights that you derived from the data. So putting a decision into action could be something like a pricing change, killing a feature, or moving a feature to a different plan."
When put together, all of these things can drive growth:
"This whole thing is essentially data-powered growth. It's not just about driving insights, making decisions, activating the data, or running experiments. It's a combination of these things."
The combination of these components is best represented in the Good Data to Growth (GDG) model. Arpit advises that companies adopt this model if data-powered growth is a priority.
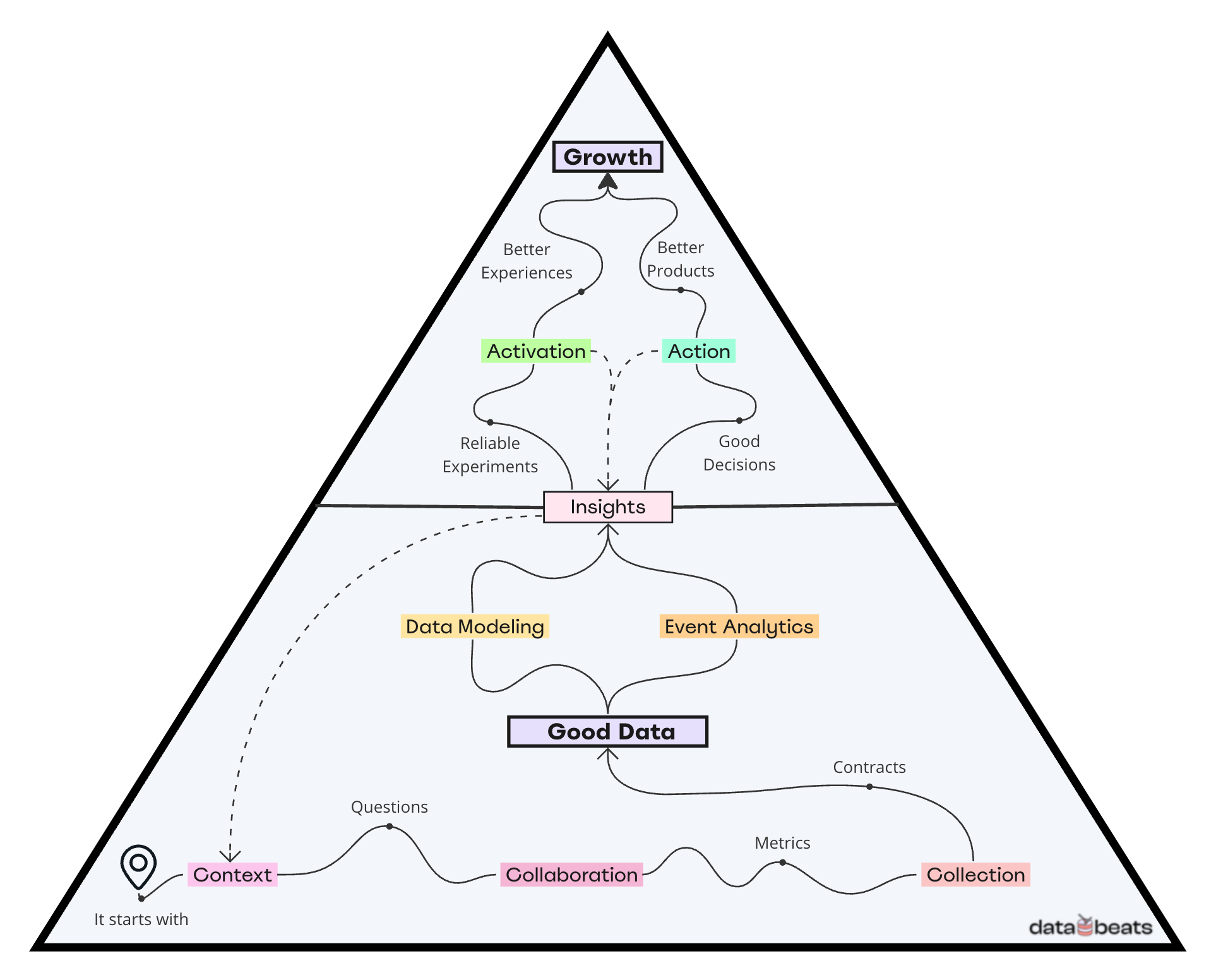
Leveraging data for business growth
Unlike product-led growth, that relies on viral loops to acquire more users, data-powered growth uses the generated data to drive business growth.
Business growth here means more revenue and more efficiency:
"You can't just rely on viral loops because there has to be more to it. You have to get users to keep using your product so they can explore new cases. This will get them to expand into different products or other features that you offer."
A robust data foundation makes good data
While good data typically means data of good quality, Arpit says it's not only about quality:
"Good quality data has a lot of attributes like accuracy, completeness, validity, and so forth.
But the way I describe good data is basically when you have a robust data foundation. It's not just about the data itself, the infrastructure, or the tooling but a combination of three main components."
A robust data foundation has three main components:
- Context
- Collaboration
- Collection
Context
"The idea is that when you have context, then you know what you want to do with the data before you actually collect the data.
You have a use case for why you want to collect a certain data point and what you're going to do with it. This helps you understand where the data originates and what is the best or the most efficient way to collect that data."
Collaboration
"This then leads to better collaboration with your data and engineering teams. And we're talking about the growth, marketing, and product people who would have some context about why they want to collect certain data, which leads to better collaboration."
Collection
"When great collaboration happens, data collection becomes really easy. The process becomes faster and you end up with good quality data."
How to develop your data tracking plan
For companies who are still developing their data tracking plan, Arpit's advice is to have context, at least three burning questions, and then decide what data to collect.
"If you don't have context, you'll be like, 'we should collect everything,' or 'we should collect these 20 events and then we'll figure out what to do with it.' Whereas the ideal process would be to first know what exactly you want to do. That's what I refer to as burning questions."
How to ask burning questions
"These questions will help you put certain data points into action."
Arpit says that burning questions are questions that have the context baked in:
"For example, 'we are acquiring a ton of users every day, but very few end up hitting the activation milestone.' That is the context. 'What's preventing the users from performing the actions that lead to activation?' That's the question."
Because without providing the context, you'll find that there could be a ton of reasons:
"If you're just asking, 'why aren't users getting activated?' There could be a hundred different reasons. Someone might say, 'maybe you're not acquiring enough users,' or 'maybe you're not acquiring the right users.'"
By asking the question with context, you can focus on what data you should collect:
"But when there's context like, 'Hey, we're acquiring a lot of users and we're able to activate a certain percentage of those users, but the rest are not activating. So what's preventing them from building that first intake scenario integration and running it right?'
That's a question that you can ask and the answer is not straightforward. To figure out the answer, you need certain data you need to understand."
But what if we don't have enough data?
If you're worried that you might not have enough data, Arpit says you can start off with a few data points. It also helps if you think of this as a continuous process:
"First, know exactly what you're going to do with the data. Have like one or two use cases, and then collect the data. Implement those use cases and learn from those use cases.
By then, you'll already have many more questions and you'll probably know what data you want to collect. Then you collect more data points and put those into action."
Setting up the right environment for data-powered growth
For many startups, it could be challenging to set up and do the first iteration of your data tracking plan because of some resistance in many aspects.
So how do you overcome these challenges?
Don't assume that data and engineering teams have background knowledge
Arpit says that the answer lies in fixing the dynamics between your data adjacent teams and your data and engineering teams so they could better collaborate:
"As a product or growth person, it really starts by understanding the workflows and the language of data and engineering teams. It's also understanding that these teams don't necessarily speak the same language."
When he was with Integromat, Arpit worked with two software engineers to implement data workflows and realized that he took a lot of things for granted:
"I took for granted that as software engineers, they would understand all the data engineering workflows, but that wasn't the case. They didn't really have any data engineering background. They also didn't know why we wanted certain data or why we're collecting certain data with so much precision."
Give them context
"Start educating them and telling them why you want certain data or what you're going to do with it.
That will help them understand how you'll use the data to drive growth and that can be additional motivation for your engineering teams to actually work with you more closely."
Don't treat data and engineering teams as service desks
Arpit says the reason why companies struggle with this is because they fail to bring everyone on the same page:
"They struggle because either there's a disconnect between the data adjacent team and the engineering team where one treats the other as like a service desk where they just make requests without really providing much context. So there's no opportunity to go into discussions, go through the process of figuring out the best way to collect this particular piece of data, or should we even collect this particular piece of data for what they're trying to do."
Other factors
Aside from bringing everyone on the same page, team structures, company culture, and semi-technical talent also play big roles in creating the right environment:
"The talent that people have in the sense that whether people are truly hungry to drive growth or not, also play a big role. There are people who really want to drive growth will do anything and everything they can to make it happen."
The insights layer of the GDG pyramid
The layer has two components: event analytics and data modeling.
Event analytics
"With product analytics tools, you can analyze the events that are taking place in your platform using funnels or different charts. It's really useful to determine where users are getting stocks, where they're dropping off, and things like that."
Data modeling
Arpit refers to the analytic data modeling in his GDG pyramid where you derive insights from the data you already have.
"Not all questions can be answered using funnel analysis. You might want to answer a question that will involve combining data from multiple sources.
In a typical data modeling process, an analyst can bring in data from multiple sources, write an SQL query (essentially a data model), and help you derive insights."
Don't collect data if you don't know what to do with it
"Every time I'm tempted to collect a data point, I ask myself, 'what am I going to do with it? How am I going to put it into action?' And if I don't have a concrete answer, I will not do it."
This principle also guides you on what to do with data privacy:
"It's also good from a privacy perspective to not collect data that you don't know what you're going to do with."
And it also helps with improving data quality:
"A lot of people think that, 'okay, let's collect all this data because we will need it in the future.' What they don't realize is that by the time the future comes, that data becomes pretty useless because the context has changed completely. It's either the product has changed, or the priorities have changed, or something else has changed."
Final advice
Do collect less data.
"Know exactly what you're going to do with it before you collect it."
Don't fall for the notion of collecting and storing all the data.
"A lot of companies today, especially those that sell data tools, are spreading this notion that you need to collect all the data from all the sources. And they're all in one place in a data warehouse because that's when you'll have a single source of truth. It's pretty useless to do that unless you know exactly what you're going to do with it."
Thanks for listening! If you found the episode useful, please spread the word on Twitter mentioning @userlist, or leave us a review on iTunes.